Artificial Intelligence Bias
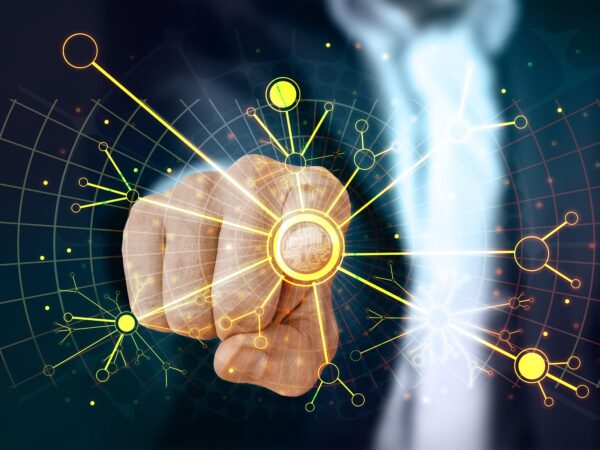
Ethical aspects of technology, such as bias in artificial intelligence, are not only quite interesting but important.
If technology, especially artificial intelligence, is so powerful we should not expand our current issues to machines because it’ll expand them. Racism, for instance, as detrimental as it is in normal life, can only get increasingly worse if we spread these biases into machines.
For instance, some people and companies create impactful algorithms where the machines are unfortunately trained on datasets lacking diversity.
So not only is there a lack of diversity in the fields of people working in AI, but the products and projects themselves as well. Now that proposes issues beyond the industry because we are all exposed to AI at this point.
Examples of fields that have been impacted by algorithmic bias:
- Facial Recognition
- Job Market
- Healthcare
- Criminal Justice system
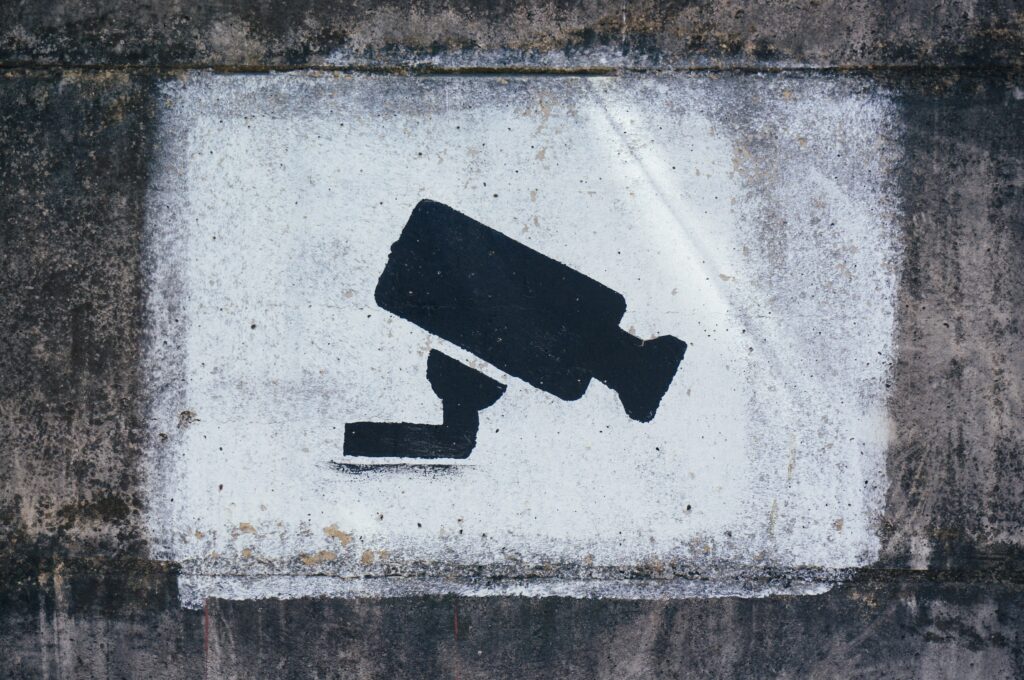
Below, I explain two failed scenarios that were particularly interesting. There are, of course, more biases beyond the ones addressed here.
- Related: Artificial Intelligence in Business
First Known Man Falsely Arrested
For over 20 years facial recognition has been used in law enforcement. Studies show that the technology is less accurate for people that are not white because of diversity lacking in the images used to train the models.
Due to a flawed match on a facial recognition algorithm on June 20, 2020, a man named Robert Julian-Borchak Williams was falsely arrested.
This was the first known account of an American being falsely matched using facial recognition, and he was held in jail for some hours. So, how many more people have been falsely identified due to the bias in certain artificial intelligence algorithms?
The criminal justice system itself has demonstrated difficulties for people of color, and to add on artificial intelligence, amplifies this issue. This scenario may not necessarily raise a completely novel issue, which is perhaps worse, because it increases an issue we already have and are having a difficult time repressing.
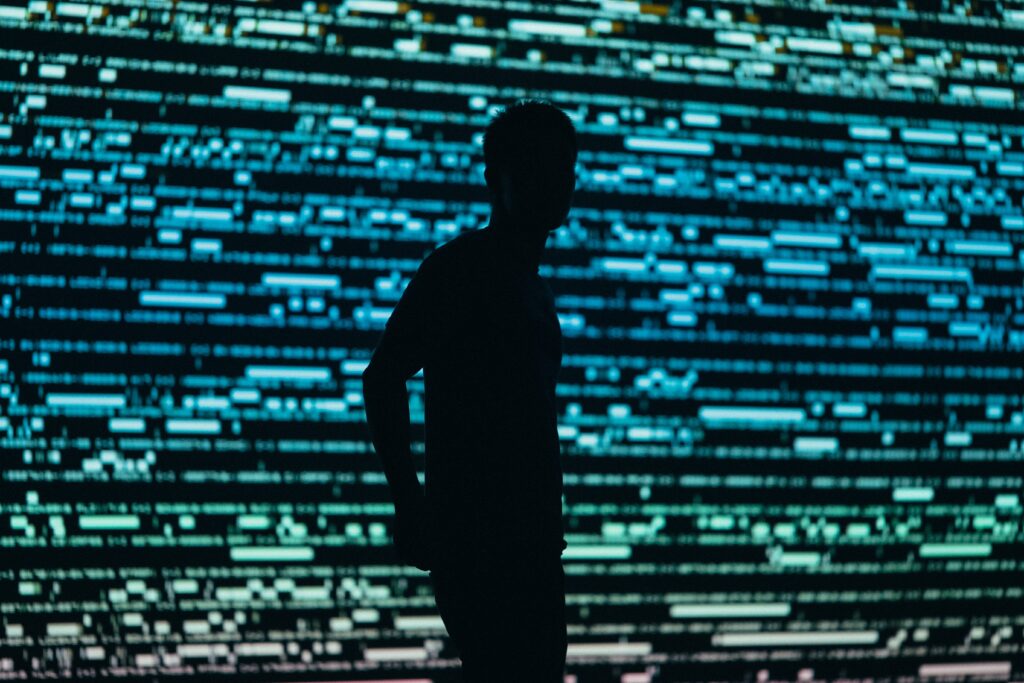
One ethical consideration is who would be at fault in Robert’s situation.
In his eyes at that moment of being falsely arrested, perhaps the policemen were at fault. In the grand scheme perhaps the engineers that developed the facial recognition software were at fault. Or the law that allowed the innovation and didn’t implement anything against such bias. Or the law enforcement that agreed to employ it and blindly trusted it (the police didn’t tell Robert why he was being arrested).
Regardless, there is a lot of social inequality in our world. So we should be wary of engineering tools that amplify that even if they’re inherently meant to help.
Amazon’s Bias Recruiting
Even leaders in tech such as Amazon couldn’t account for their bias. The company wanted to automate its hiring process by finding the top people to hire in its large pile of job applicants. They did this by developing an AI that could find the top 5% of applicants. It trained and learned patterns on the set of top past employees.
The problem was, that all those people being trained on were, due to societal factors back then, primarily white males.
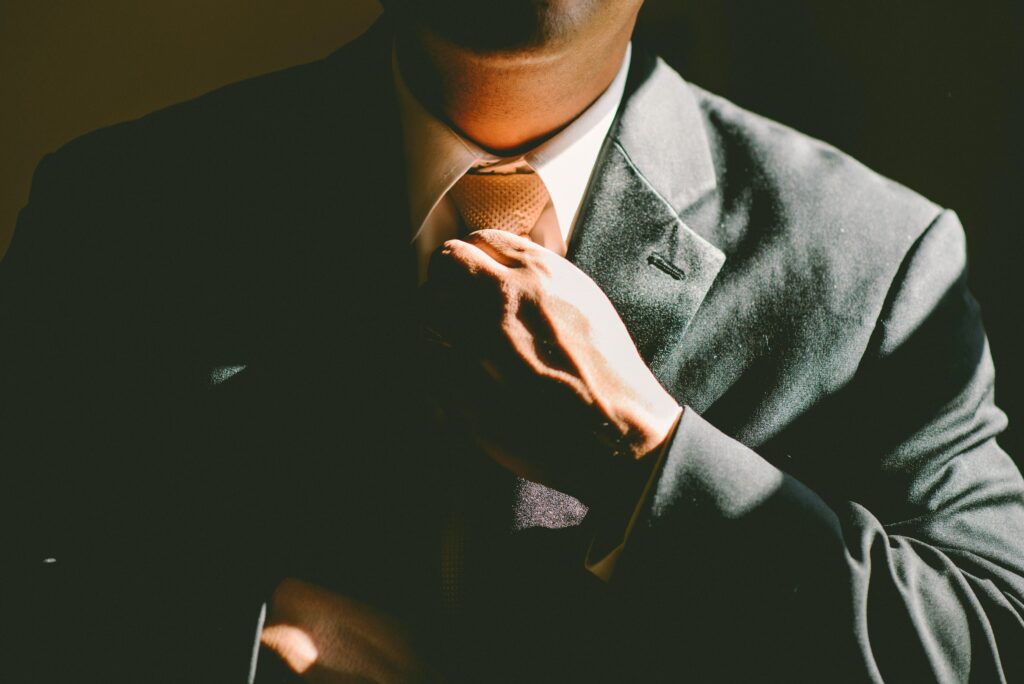
So, due to its training data it was biased towards males. And the model learned that anything associated with women corresponded with a bad applicant.
If, for instance, you had a name associated with women, you’d be significantly less likely to be a top applicant, severely diminishing your chance at a job at Amazon.
Study on Gender Bias
These stories aren’t just a couple of coincidences. There have been studies evidencing that artificial intelligence algorithms have biases against people of color, especially women.
Gender Shades, by MIT student, is a great example, you can watch a video explaining it clearly here.
The study shows how IBM, Microsoft, and Face++ had good overall accuracy but had error differences when it came to genders and skin tones.
The order of accuracy demonstrated (from most to least): lighter men, lighter females, darker men, darker females. The largest gap in accuracy from IBM: 34.4% between lighter males and darker females.
Future of Bias in Artificial Intelligence
An important path to go about this would be to learn the ethical implications of your work, to act responsibly, and have the next generation of teachers teach an ethical approach.
Also, to implement more regulations towards bias, and not let it slip as a lack of importance. As Embedded EthiCS students, for example, learn don’t think “Can I build it?”, but “Should I build it, and if so, how?”.